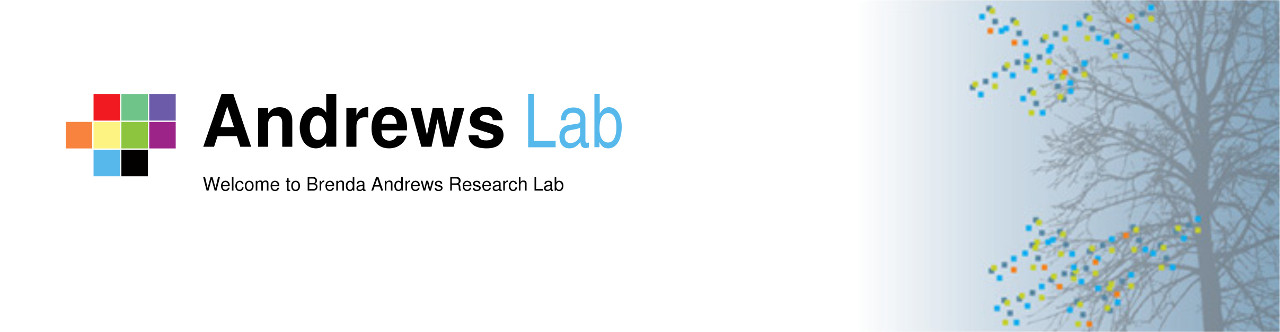
Projects
We are interested in exploring the genotype to phenotype relationship in budding yeast and human cells using high content screening, functional genomics and computational analyses.
Biological Discovery Using Imaging
Identifying Subcellular Morphology Defects for 18 Compartments in All Mutants
Nil Sahin
One of the great methods to understand gene function is perturbing the gene and identifying the phenotypic consequences for the cell by imaging. We have now done imaging screens of all ~6000 deletion and temperature-sensitive mutants expressing 18 fluorescently tagged proteins from different compartments. For such an enormous dataset, we have developed image analysis methods to automatically detect cells with abnormal morphologies, based on outlier detection. This detection allows us to quantify the percentage of cells with abnormal phenotypes for a perturbed gene population, i.e. the penetrance. We have combined outlier detection and a neural network-based image analysis of single cells to quantify the phenotypic variability within a population of cells and are classifying mutant phenotypes for all mutants for all 18 compartments. This study will further our understanding of the regulation of cellular morphology.
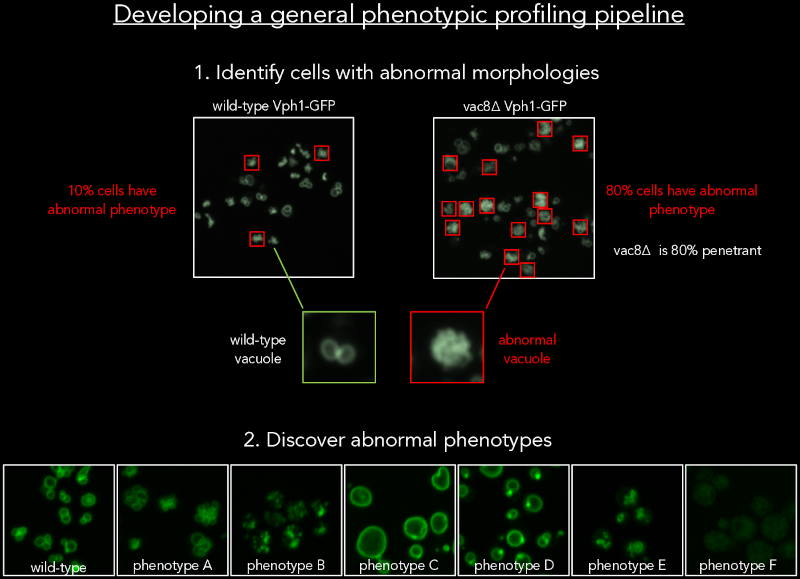
Understanding How Cells Die
Dara Lo
Understanding how cell die is important both for keeping them alive and for promoting cell death in situations where that is important, but this topic has not been well explored. To study the pattern of subcellular events that occur as cells die, we are using 350 temperature-sensitive mutants of essential genes, each expressing one of 20 different GFP-tagged proteins. By imaging cells over a time course at nonpermissive temperature, we are uncovering the order of events that occur as cells die. We find that similar terminal phenotype trajectories tend to occur in mutants with defects in related bioprocesses. Comparison with wild-type cells dying of old age, in collaboration with the Nystrom lab (University of Gothenburg), gives us further insight into replicative aging.
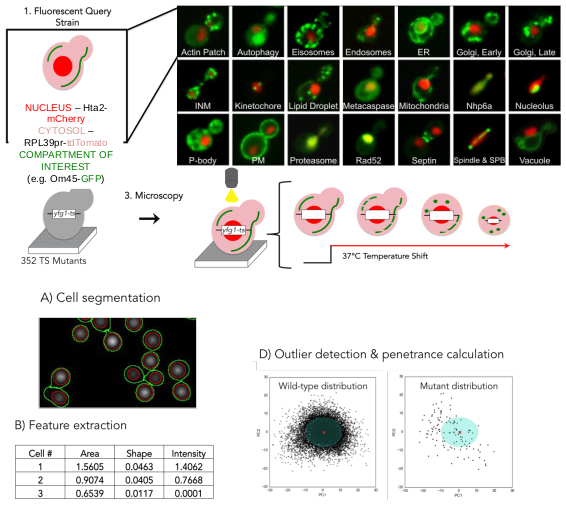
Understanding How Cells Handle Misfolded Proteins
Kyle Wang
Protein homeostasis is linked to many neurodegenerative diseases including Parkinson’s, Huntington’s and Alzheimer’s. To understand how the cell deals with different misfolded proteins, we are constructing a collection of hundreds of temperature-sensitive (TS) alleles of essential genes tagged with GFP. Despite their well-established utility, the dynamics of the mutant proteins encoded by TS alleles remain unexplored. By coupling the power of high throughput yeast genetics and high content screening, we are systematically uncovering the genetic networks and molecular mechanisms governing protein misfolding and quality control in different subcellular compartments.
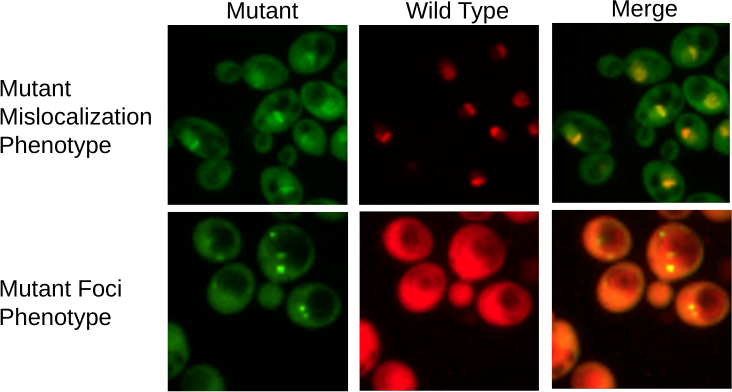
Defining the Spatiotemporal Proteome of the Eukaryotic Cell Division Cycle
Athanasios Litsios
The execution of the cell division cycle relies heavily on intra-cellular protein movements that mediate regulatory functions and serve cellular logistics. Currently, we are lacking a proteome-level view of changes in protein localization as a function of cell cycle progression. We have co-measured, at the proteome-level, changes in protein localization and concentration in live single cells during the execution of the cell division cycle, combining systematic genetics and deep learning. In this way, we have generated a high-resolution, spatiotemporal map of the eukaryotic cell division cycle. By complementing our single-cell data with transcript and translational efficiency measurements, we are able to dissect forces that shape cell cycle-related proteome periodicity.
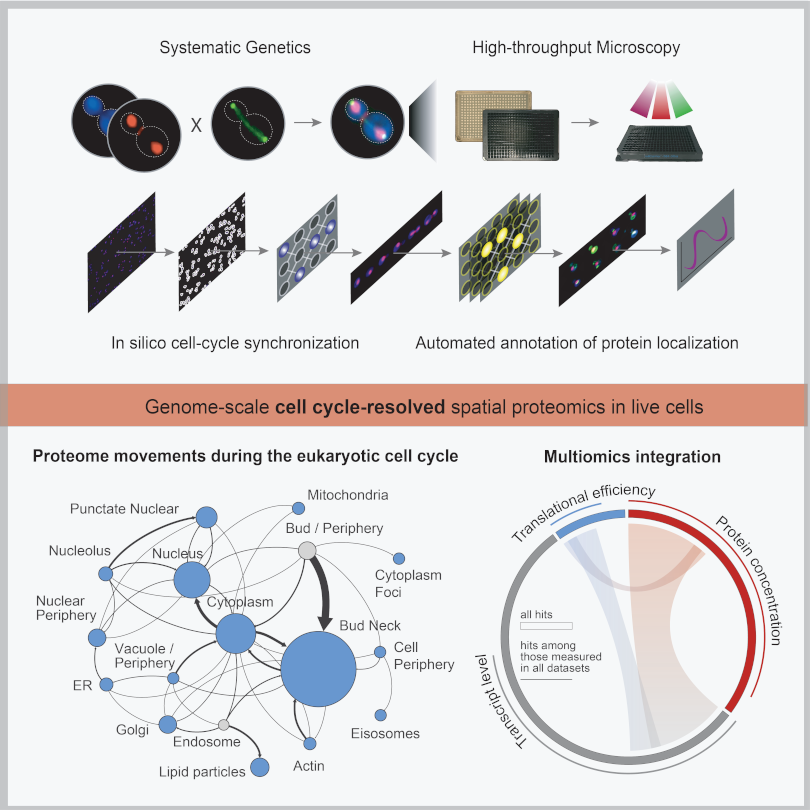
Systematically Quantifying Proteostasis in Live Single Cells
Maintenance of proteome homeostasis is pivotal for proper cellular function. We combine HTP-genetics and -microscopy to understand the forces that shape the composition, abundance, and localization of the proteome in single cells. Since proteome abundance is a product of the combined changes in protein production and degradation rate, we are developing a fluorescence-microscopy-based platform for systematic, concomitant measurement of protein abundance, production, degradation, and localization, in live yeast cells. This effort is expected to reveal fundamental principles of proteome homeostasis in eukaryotic cells.
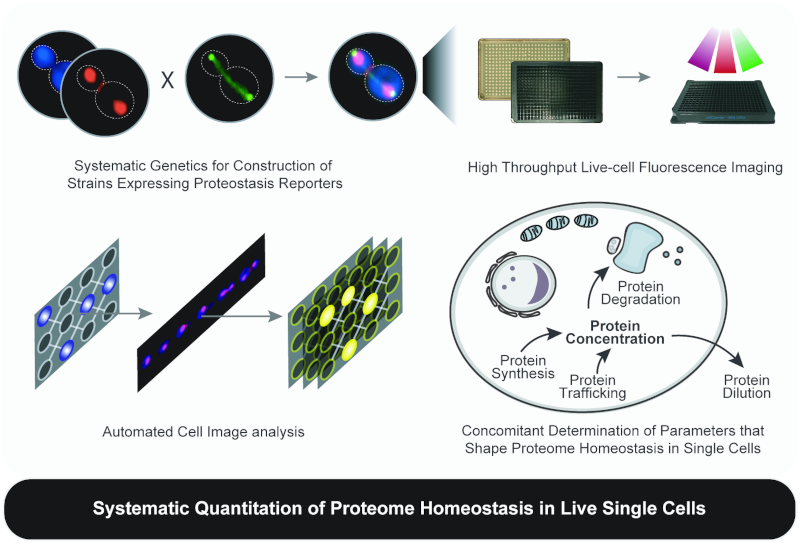
Genetic Interactions in Human Cells
Global Mapping of Human Genetic Interactions in HAP1
We are using the CRISPR-Cas9 system with the lentiviral Toronto knockout library (KOv3) to systematically introduce mutations in a ~haploid human cell line using single-guide RNAs (sgRNAs) in a pool, allowing the collection of large-scale GI data in human cells.
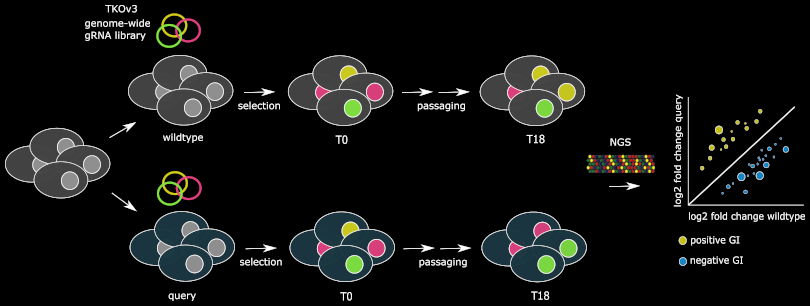
Mapping Genetic Interactions of Human Essential Genes
Jason Wang
In yeast, we found that hypomorphic alleles of essential genes have substantially more GIs than deletions of non-essential genes. We are using several approaches to systematically create hypomorphic alleles of human essential genes and cataloguing a collection of stable cell lines. These are then used as queries to screen for genetic interactions via a genome-wide CRISPR-Cas9 knockout method.
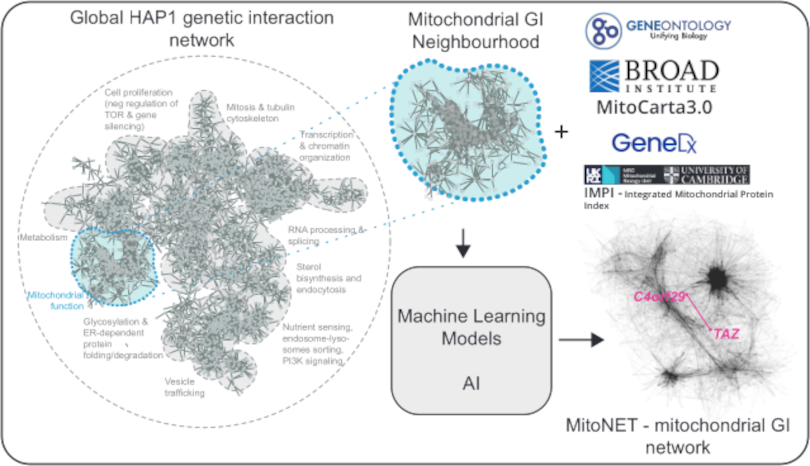
Generating A Mitochondrial Network in Health and Disease
Sanna Masud
Genetic interactions (GIs) occur when mutations in multiple genes combine to generate an unexpected phenotype, and aid in our understanding of genotype-to-phenotype relationships in both health and disease. Barth Syndrome (BTHS) is an X-linked recessive syndrome caused by mutations in the gene TAZ, which encodes a highly conserved mitochondrial acyltransferase (Tafazzin) involved in cardiolipin biogenesis. There is extreme variability observed in Barth Syndrome patients, even though one known gene is responsible for this disease, suggesting that there are modifier genes or additional variants of TAZ that could be involved. Interestingly, very little is currently known about the TAZ gene and the spectrum of functions it may be involved with in the cell. We are generating the first GI profile of the TAZ gene and, in parallel, a comprehensive reference GI network of mitochondria-related genes in human cells, exploring relevant disease alleles and GIs involved in both physiological and pathological conditions. Together, these data will contribute to the human GI network project, and further our understanding of both the TAZ gene and mitochondrial function.
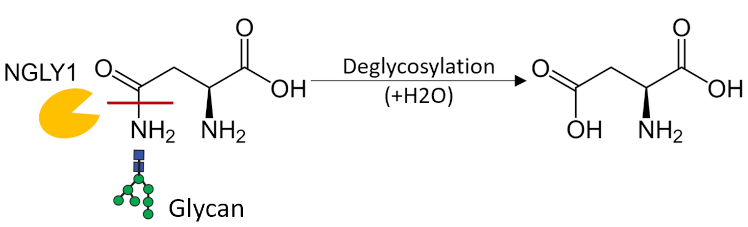
Exploring the Biological Role of a Deglycosylase Implicated in a Rare Disease
Sylvia Almeida
NGLY1 Deficiency is a rare multi-systemic autosomal recessive disease caused
by mutations in N-glycanase 1 (NGLY1) and has no known cure. Patients with the disease present
with a broad range of symptoms including developmental delays, hypotonia, intellectual and motor
impairments, liver dysfunction, and seizures. NGLY1 is a deglycosylase which has been implicated
in protein quality control and regulation of the proteasome. However, much is still unknown
about its biological role in the cell and the mechanisms of disease pathogenesis are poorly
understood.
Our goal is to elucidate the function of NGLY1 in human cells. One known role of NGLY1 is in
activating the response to proteotoxic stress and NGLY1 mutant cells are hypersensitive to
proteasome inhibition. Therefore, we aim to investigate the mechanisms by which cells can become
resistant to proteotoxic stress to identify pathways which can suppress the proteostatic defects
caused by loss of NGLY1. A second aim of this project is to survey NGLY1 function on a global
scale. To achieve this, we are using genome-wide CRISPR-Cas9 screening of the nearly haploid
human cell line HAP1 as an unbiased strategy to profile NGLY1 and potentially identify novel
roles for this gene in the cell.
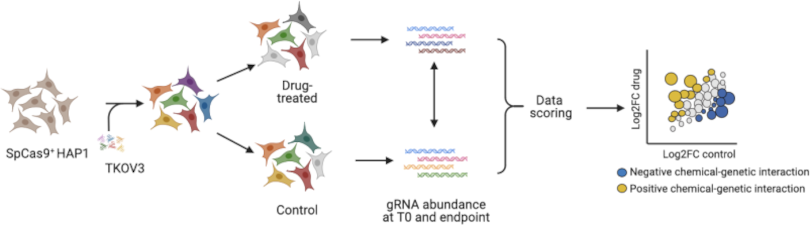
Functional Characterization of Chemical-Genetic Interactions
Urvi Bhojoo
We are screening for chemical genetic interactions using the CRISPR-Cas9 system and the Toronto knock-out library of gRNAs. Screening a set of mutant strains against a library of compounds will allow us to uncover their modes of action and discover new potential therapeutics and chemical probes.
Complex Genetics in Budding Yeast
Environmental Robustness of the Global Yeast Genetic Interaction Network
Vincent Messier
Phenotypes associated with genetic variants can be altered by interactions with other genetic variants (GxG), with the environment (GxE), or both (GxGxE). We previously mapped yeast genetic interactions on a global scale, but the environmental influence on the plasticity of genetic networks had not been examined systematically. To assess environmental rewiring of genetic networks, we examined 14 diverse conditions and scored 30,000 functionally representative yeast gene pairs for dynamic, differential interactions. Different conditions revealed novel differential interactions, which often uncovered functional connections between distantly related gene pairs. However, the majority of observed genetic interactions remained unchanged in different conditions, suggesting that the global yeast genetic interaction network is robust to environmental perturbation.
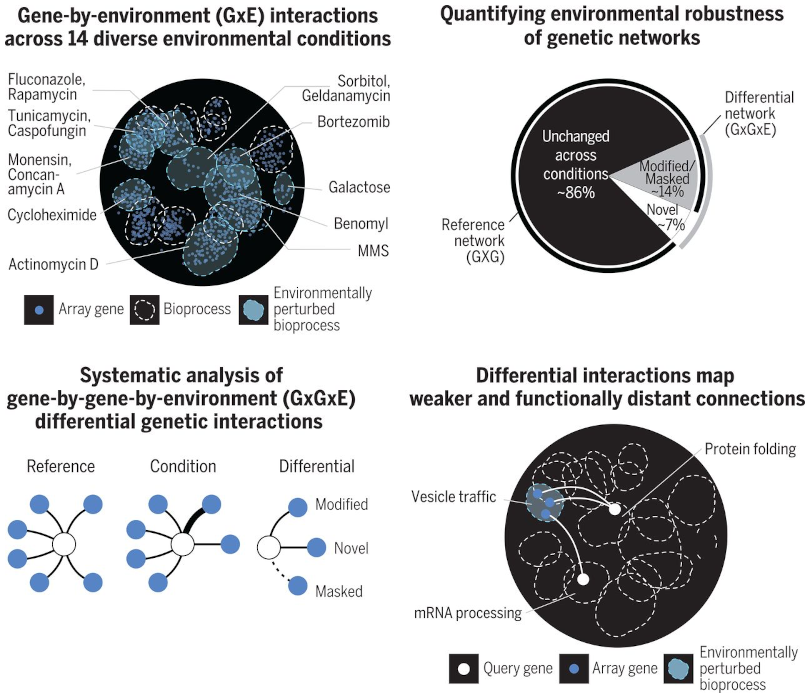
Complex Haploinsufficiency of the Yeast Essential Genome
Thuy Nguyen
A fitness defect from hemizygosity is known as simple haploinsufficiency, whereas complex haploinsufficiency (CHI) occurs when hemizygosity at two or more loci in a diploid cell confers a fitness defect. This type of interaction is likely to explain some disease phenotypes in humans, who are obligate diploids. Using automated yeast genetics, we are screening for CHI interactions in the Saccharomyces cerevisiae essential genome.
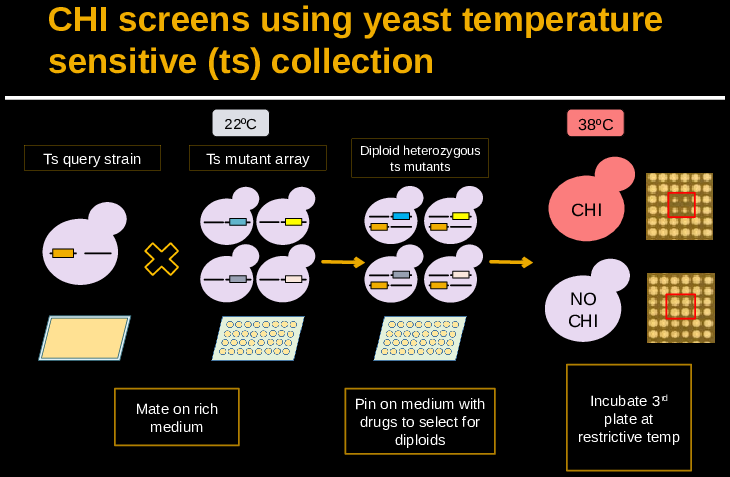
Identifying Bypass Suppressors of Synthetic Lethal Gene Pairs
Clarence Yeung
Genetic suppression occurs when a mutation in a gene can compensate for the fitness defect of another gene that is required for viability. Advances in genetic sequencing have identified causal variants for Mendelian disorders; however, a subset of individuals with these mutations have little or no phenotype due to secondary site mutations that confer a protective effect. Mapping out these relationships represents a way to understand the wiring of the genome, as well as identifying ways to bypass it. Although technologies such as CRISPR-Cas9 has facilitated genome editing in mammalian cells, doing this systematically and on a genome-wide scale remains laborious and expensive. We are using the model organism, Saccharomyces cerevisiae, to study genetic suppression using a high-throughput pipeline to systematically identify suppressors of synthetic lethal gene pairs. This involves the creation of a set of query strains that can be kept alive using a plasmid-based system, followed by Synthetic Genetic Array (SGA) technology to systematically introduce mutations. Compared to traditional methods of isolating and identifying suppressors through genetic crosses and sequencing, this approach can exhaustively and unambiguously identify monogenic suppressors. Mapping a network of suppressors of synthetic lethal gene pairs will illuminate mechanisms by which a cell is able to tolerate genetic insults and further characterize the functional wiring of a cell.
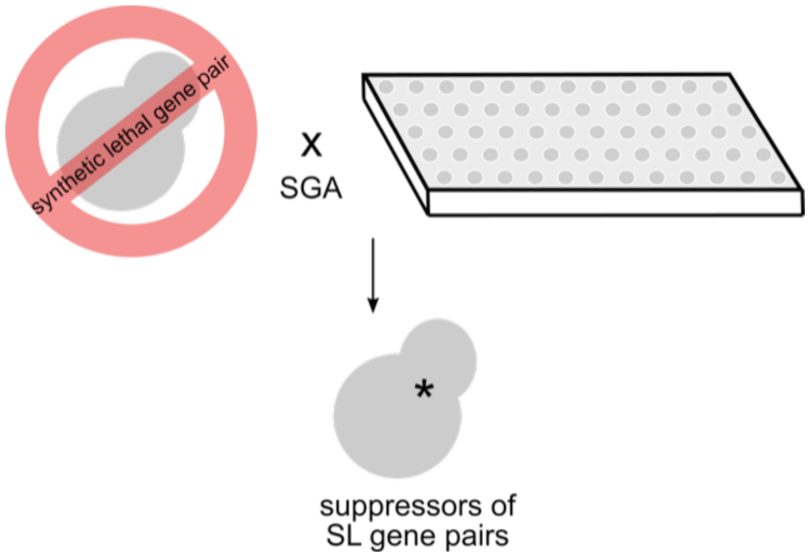
Constructing New Yeast Collections to Discover Novel Biology
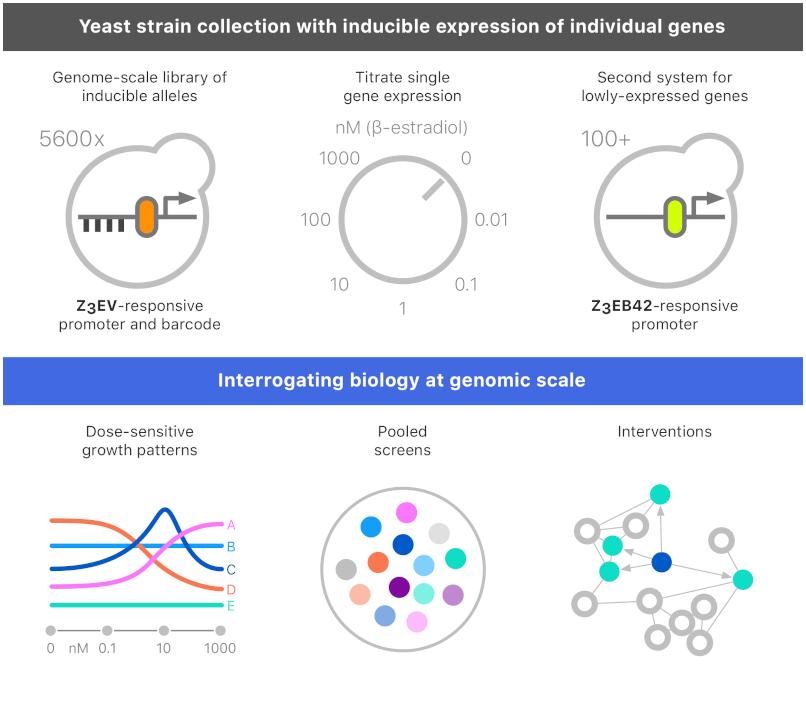
The YETI Collection (Yeast Estradiol strains with Titratable Induction)
The ability to switch a gene from off to on and monitor dynamic changes provides a powerful approach for probing gene function and elucidating causal regulatory relationships. We have constructed the YETI (Yeast Estradiol strains with Titratable Induction) collection, in which >5,600 yeast genes are engineered for transcriptional inducibility with single-gene precision at their native loci and without plasmids. Each strain contains SGA screening markers and a unique barcode, enabling high-throughput genetics. We have characterized the YETI collection using growth phenotyping as well as SGA and BAR-seq screens.
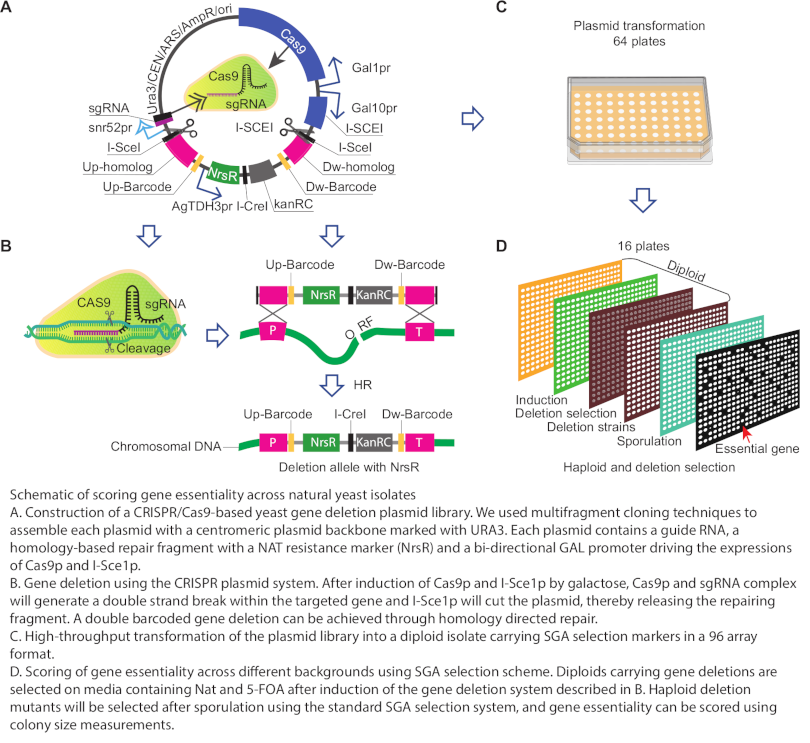
Scoring Gene Essentiality across Natural Yeast Isolates
Guihong Tan
Different wild yeast strains are specialized for different niches, which is reflected by their different genetics. To gain comparative insights into background-dependent fitness phenotypes and associated modifier interactions, and to dissect the core essential genes of yeast requires the generation of systematic deletion mutant collections in genetically diverse strains. Traditional PCR-based is costly and can be a major bottleneck at this scale. We have developed a collection of CRISPR-Cas9 based gene deletion plasmids consisting of ~5800 individual plasmids (> 98% of the genome), each of which targets and deletes a unique gene in the yeast genome through homology-based repair. These plasmids are designed to take into account the sequence divergence among different wild strain backgrounds, such that the guide RNA can target every strain in our collection despite their genome diversity, and the repair fragments include large homology regions of ~200 bp ensuring efficient repair in any background. We can score gene essentiality across natural yeast isolates individually in an array format. In addition, deletion mutants generated with these plasmids carry unique 20 bp DNA barcodes, which allows for their identification using a pooled barcode sequencing strategy. With these resources, we are focusing on ~10 diverse genetic backgrounds to generate systematic gene deletion collections.
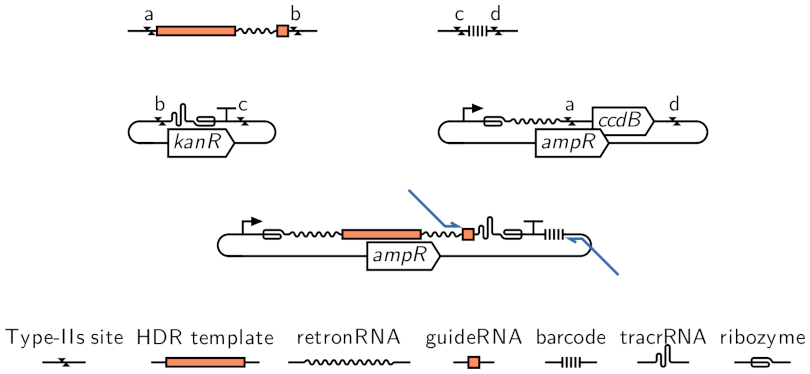
CRISPR-based Tools to Generate and Phenotype Pooled Collections of Yeast Mutants
José Rojas Echenique
A central aim of evolutionary biology is the development of models capable of predicting the effects of mutations. Towards this aim, huge collections of mutants have been screened, providing a first glimpse into the genotype to phenotype map. Since the effects of a mutation depend both on other mutations in the genome, and on the ecological context of the organism, tools to generate ever larger collections of mutants in diverse genetic backgrounds and to assay their phenotypes in more contexts are of critical importance. We are developing CRISPR-based tools to generate and phenotype pooled collections of yeast mutants using array-synthesized oligos.
Computational Analyses
Integrating Different Datasets
Duncan Forster
We work on integrating many diverse biological networks (such as protein-protein interaction and genetic interaction networks) to generate high quality and high coverage functional descriptions of genes. Network integration allows us to capture relevant functional information from multiple data sources - increasing the robustness of our downstream gene function annotations and improving functional module detection. We have developed a deep learning algorithm called BIONIC (Biological Network Integration using Convolutions), which incorporates a recent major advancement in learning over network-structured data, namely the graph convolutional network. BIONIC can encode many large and diverse networks into a shared feature set, where each gene is associated with learned features describing the gene's relationships across the input networks. These features capture functional information, such as gene-gene relationships, and can be clustered to identify functional modules. We hope to apply BIONIC to large-scale human datatypes to generate an improved set of gene function annotations and detect new biological modules in the human cell.
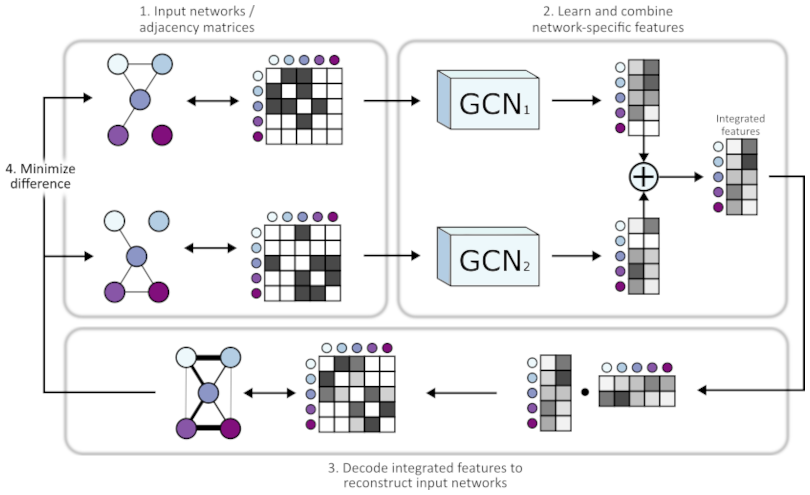
Integrating More Types of Datasets
Qi Wu
The cell map conceptually defines a comprehensive map that assembles functional genomics data to describe the functional aspect of a cell. Aiming to define and build a unified cell map, we are using deep learning approaches to integrate data from multiple perspectives, such as protein-protein interaction networks, genetic interaction networks, protein sequences, literature-mining derived textual descriptions, and proteomics imaging data.
A Deep Learning Method to Identify and Characterize Fluorescently Labelled Proteins from Live-cell Imaging
Anastasia Razdaibiedina
We have developed an unsupervised deep learning method to group proteins based on features extracted from micrographs using a convolutional neural network (CNN). Since functionally related proteins often have similar localization and morphological patterns, we can leverage this information to group proteins with similar visual profiles into similarity clusters. Unsupervised features obtained from this method reveal the hierarchical organization of a cell at different levels.
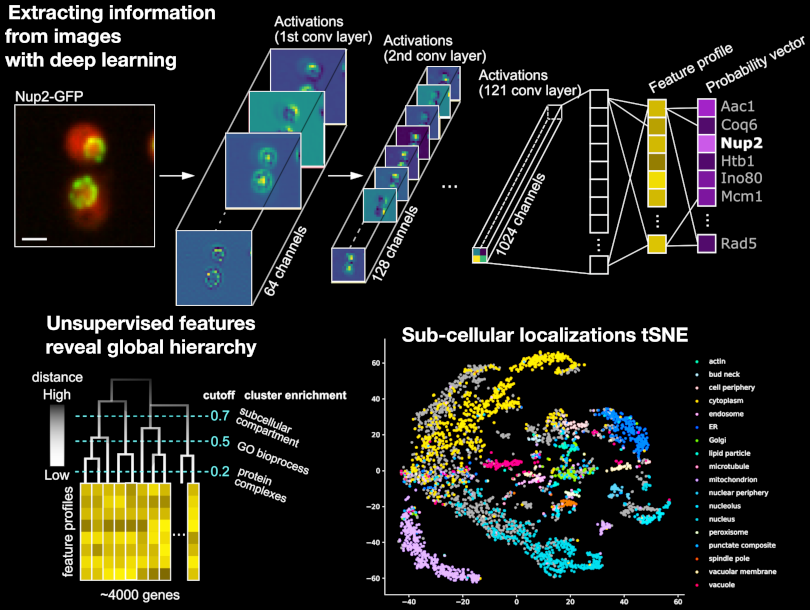